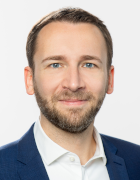
Prof. Dr. Stephan Günnemann
Academic Career and Research Areas
Stephan Günnemann conducts research in the area of machine learning and data analytics. His main research focuses on how to make machine learning techniques reliable, thus, enabling their safe and robust use in various application domains. Prof. Günnemann is particularly interested in studying machine learning methods targeting complex data domains such as graphs/networks and temporal data.
He acquired his doctoral degree in 2012 at RWTH Aachen University in the field of computer science. From 2012 to 2015 he was an associate of Carnegie Mellon University, USA; initially as a postdoctoral fellow and later as a senior researcher. Prof. Günnemann has been a visiting researcher at Simon Fraser University, Canada, and a research scientist at the Research & Technology Center of Siemens AG. In 2015, Prof. Günnemann set up an Emmy Noether research group at TUM Department of Informatics. He has been a professor at TUM since 2016. He is the Executive Director of the Munich Data Science Institute and Director of the Konrad Zuse School of Excellence in Reliable AI.
Awards
- Heinz Maier-Leibnitz Medal (2022)
- Google Faculty Research Award in Machine Learning (2020)
- ACM SIGKDD Best Research Paper Award (2018)
- Junior-Fellow of the German Computer Science Society (2017)
- Member of the Emmy Noether Program of the German Research Foundation (DFG) (2015)
Key Publications (all publications)
S Günnemann. “Graph neural networks: Adversarial robustness”. Graph Neural Networks: Foundations, Frontiers, and Applications, Springer, 2022.
AbstractJ Gasteiger, J Groß, S Günnemann. “Directional message passing for molecular graphs”. International Conference on Learning Representations, 2020.
AbstractJ Gasteiger, A Bojchevski, S Günnemann. “Predict then propagate: Graph neural networks meet personalized pagerank”. International Conference on Learning Representations, 2019.
AbstractZügner D, Akbarnejad A, Günnemann S: "Adversarial Attacks on Neural Networks for Graph Data". ACM SIGKDD Conference on Knowledge Discovery and Data Mining. 2018: 2847-2856.
AbstractBojchevski A, Shchur O, Zügner D, Günnemann S: "NetGAN: Generating Graphs via Random Walks". International Conference on Machine Learning. 2018; 609-618.
AbstractIf you wish your profile to be changed or updated please contact Franz Langer.