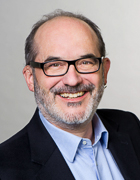
Prof. Dr.-Ing. Klaus Diepold
Professur
Kontakt
Wissenschaftliche Laufbahn und Forschungsgebiete
Prof. Diepold (*1961) forschte im Bereich Multimedia-Signalverabeitung mit Schwerpunkt beim Entwurf schneller Algorithmen. Heute konzentriert er sich auf das maschinelle Lernen, u.a. für die Entwicklung moralischer Maschinen und Modellierung und Simulation von Affekten für kognitive Systeme. Am Center for Digital Technology and Management (CDTM) engagiert er sich für die Entrepreneurship-Ausbildung Studierender aller Fachrichtungen.
Nach Studium und Promotion (1992) der Elektro-und Informationstechnik an der TUM entwickelte Prof. Diepold Video-Kompressionssysteme und MPEG-Standards (MPEG-4, MPEG-A). 2002 folgte der Ruf an den Lehrstuhl für Datenverarbeitung der TUM. 2005–10 engagierte er sich als Studiendekan. Gastprofessuren führten ihn nach Alberta, Kanada und zu NICTA in Australien. Er ist Mitglied im Aufsichtsrat des CDTM und war 2006–14 im Vorstand des Exzellenzclusters Cognition for Technical Systems (CoTeSys). Von 2013–15 war Prof. Diepold Vizepräsident für Diversity und Talent Management. 2018 ging die von ihm initiierte Webserie „Technically Single“ national auf Sendung.
Wichtigste Auszeichnungen
- Fellowship für Innovation in der Hochschullehre, Stifterverband für die Deutsche Wissenschaft (2012)
- Certificate of Appreciation, ISO/IEC for work on MPEG-A (2008)
- TUM Lehrpreis (2004)
- Award for most innovative software product for “MotionPerfect” (2001)
- Best Paper Award Society for Information Technology (ITG) (1992)
Schlüsselpublikationen
M Kissel, K Diepold. Structured Matrices and Their Application in Neural Networks: A Survey. New Generation Computing 41 (3), 697-722, 2023.
AbstractLJ Meier, A Hein, K Diepold, A Buyx. Algorithms for ethical decision-making in the clinic: A proof of concept. The American Journal of Bioethics 22 (7), 4-20. 2022.
AbstractS Hawe, M Kleinsteuber, K Diepold. Analysis operator learning and its application to image reconstruction. IEEE Transactions on Image Processing 22 (6), 2138-2150. 2013.
AbstractS Gronauer, K Diepold. Multi-agent deep reinforcement learning: a survey. Artificial Intelligence Review, 1-49. 2022.<o:p></o:p>
AbstractT Hoßfeld, C Keimel, M Hirth, B Gardlo, J Habigt, K Diepold, P Tran-Gia. Best practices for QoE crowdtesting: QoE assessment with crowdsourcing. IEEE transactions on multimedia 16 (2), 541-558. 2013.
AbstractBei Änderungs- oder Aktualisierungswünschen wenden Sie sich bitte an Franz Langer.