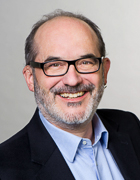
Prof. Dr.-Ing. Klaus Diepold
Academic Career and Research Areas
Professor Diepold (*1961) conducted research in the field of multimedia signal processing with a focus on the design of fast algorithms. Today, he concentrates on machine learning, which entails developing moral machines as well as modeling and simulating affects for cognitive systems. He is also actively involved in entrepreneurship training for students from all departments at the Center for Digital Technology and Management (CDTM) and promotes entrepreneurship within his own department.
After studying electrical engineering and information technology and completing his PhD (1992) at TUM, Professor Diepold developed video compression systems and MPEG standards (MPEG-4, MPEG-A) in industry. In 2002, he accepted the Chair of Data Processing at TUM, where he served as Dean of Studies from 2005–10. Visiting professorships took him to Alberta, Canada and NICTA, Australia. Prof. Diepold is a member of CDTM’s supervisory board and was on the board of management of the cluster of excellence Cognition for Technical Systems (CoTeSys) from 2006-14. From 2013–15, Professor Diepold served as TUM's Senior Vice President for Diversity and Talent Management. In 2018, the web video series “Technically Single” which he had initiated aired nationwide.
Awards
- Fellowship for Innovation in University Teaching, Stifterverband für die Deutsche Wissenschaft (2012)
- Certificate of Appreciation, ISO/IEC for work on MPEG-A (2008)
- TUM Teaching Prize (2004)
- Award for most innovative software product for “MotionPerfect” (2001)
- Best Paper Award Society for Information Technology (ITG) (1992)
Key Publications
M Kissel, K Diepold. Structured Matrices and Their Application in Neural Networks: A Survey. New Generation Computing 41 (3), 697-722, 2023.
AbstractLJ Meier, A Hein, K Diepold, A Buyx. Algorithms for ethical decision-making in the clinic: A proof of concept. The American Journal of Bioethics 22 (7), 4-20. 2022.
AbstractS Hawe, M Kleinsteuber, K Diepold. Analysis operator learning and its application to image reconstruction. IEEE Transactions on Image Processing 22 (6), 2138-2150. 2013.
AbstractS Gronauer, K Diepold. Multi-agent deep reinforcement learning: a survey. Artificial Intelligence Review, 1-49. 2022.<o:p></o:p>
AbstractT Hoßfeld, C Keimel, M Hirth, B Gardlo, J Habigt, K Diepold, P Tran-Gia. Best practices for QoE crowdtesting: QoE assessment with crowdsourcing. IEEE transactions on multimedia 16 (2), 541-558. 2013.
Abstract