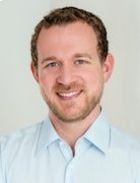
Prof. Dr. Dominik Grimm
Professur
School
Kontakt
Wissenschaftliche Laufbahn und Forschungsgebiete
Prof. Grimm (*1985) forscht auf dem Gebiet der Bioinformatik und konzentriert sich auf die Entwicklung statistischer und maschineller Lernverfahren, um komplexe biologische Systeme, ihre Funktionsweise und biochemischen Eigenschaften besser zu verstehen. Er arbeitet an Methoden zur Erkennung von Genotyp-Phänotyp-Beziehungen und zur Erfassung phänotypischer Merkmale aus Bildern. Ein besonderer Schwerpunkt liegt auf der Erforschung künstlicher Intelligenz, um Probleme in den Biowissenschaften und der Chemie ohne spezifisches Vorwissen zu lösen.
Prof. Grimm studierte Bioinformatik an der Hochschule Weihenstephan-Triesdorf (2011), mit Auslandsaufenthalten an der Universität Cambridge, dem Europäischen Bioinformatik Institut, Großbritannien und der University of New South Wales, Sydney, Australien. Er promovierte am Max-Planck-Institut (MPI) für Entwicklungsbiologie, am MPI für Intelligente Systeme und an der Universität Tübingen (2015). Nach einem Postdoc am D-BSSE der ETH Zürich (2016) und einer kurzen Zeit in der Industrie wurde er 2018 auf die Professur für Bioinformatik berufen.
Wichtigste Auszeichnungen
- Preis der Fachschaft für exzellente Lehre am TUM Campus Straubing (2021)
- Preis der Fachschaft für exzellente Lehre am TUM Campus Straubing (2020)
Schlüsselpublikationen (alle Publikationen)
Pirnay, J., Göttl, Q., Burger, J., & Grimm, D. G. (2023). Policy-Based Self-Competition for Planning Problems. In International Conference on Learning Representations (ICLR).
AbstractJohn, M., Ankenbrand, M. J., Artmann, C., Freudenthal, J. A., Korte, A., & Grimm, D. G. (2022). Efficient permutation-based genome-wide association studies for normal and skewed phenotypic distributions. Bioinformatics, 38(Supplement_2), ii5-ii12.
AbstractGenze, N., Ajekwe, R., Güreli, Z., Haselbeck, F., Grieb, M., & Grimm, D. G. (2022). Deep learning-based early weed segmentation using motion blurred UAV images of sorghum fields. Computers and Electronics in Agriculture, 202, 107388.
AbstractGrimm, D. G., Roqueiro, D., Salomé, P. A., Kleeberger, S., Greshake, B., Zhu, W., ... & Borgwardt, K. M. (2017). easyGWAS: a cloud-based platform for comparing the results of genome-wide association studies. The Plant Cell, 29(1), 5-19.
AbstractGrimm, D. G., Azencott, C. A., Aicheler, F., Gieraths, U., MacArthur, D. G., Samocha, K. E., ... & Borgwardt, K. M. (2015). The evaluation of tools used to predict the impact of missense variants is hindered by two types of circularity. Human mutation, 36(5), 513-523.
AbstractBei Änderungs- oder Aktualisierungswünschen wenden Sie sich bitte an Franz Langer.