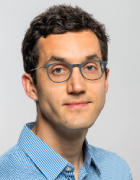
Prof. Dr. Reinhard Heckel
Professur
Kontakt
Wissenschaftliche Laufbahn und Forschungsgebiete
Reinhard Heckel ist Professor für Maschinelles Lernen am Department of Computer Engineering an der TUM (Technische Universität München). Von 2017 bis 2019 war er Tenure-Track Assistant Professor an der Rice University, US. Davor war er Postdoktorand im Berkeley Artificial Intelligence Research Lab am EECS-Department an der University of California, Berkeley, und arbeitete für ein Jahr in dem Cognitive Computing and Computational Sciences Department bei IBM Research Zürich. Er promovierte 2014 an der ETH Zürich und war Gastdoktorand Stanford University’s Statistik Department. 2022 wurde er von Capital Magazine zu Deutschlands "Top 40 unter 40" ernannt und von der Werner-von-Siemens Ring Stiftung für seine Arbeit als Jungwissenschaftler ausgezeichnet.
Reinhard Heckel's Forschung konzentriert sich auf Maschinelles Lernen und Informationsverarbeitung. Er arbeitet an der Entwicklung von Algorithmen und theoretischen Grundlagen für deep learning, insbesondere in der medizinischen Bildverarbeitung, und an der Etablierung mathematischer und empirischer Grundlagen für Maschinelles Lernen. Darüber hinaus entwickelt er DNA-Datenspeicherung und beschäftigt sich mit der Nutzung von DNA als digitale Informationstechnologie.
Wichtigste Auszeichnungen
- Capital 40 under 40 (2022)
- Auszeichung als Jungwissenschaftler der Werner-von-Siemens-Ring Stiftung (2022)
- Featured in der BBC-Future Series ‘The genius behind: This is how to store human knowledge for eternity’ (2015)
- Medaille der ETH Zürich für eine herausragende Doktorarbeit (2015)
- IBM first patent application invention achievement award (2015)
Schlüsselpublikationen (alle Publikationen)
M. Zalbagi Darestani, Akshay Chaudhari, and R. Heckel, 'Measuring robustness in deep learning based compressive sensing’, ICML 2021 (International Conference on Machine Learning) (long talk).
AbstractR. Heckel and M. Soltanolkotabi, 'Compressive sensing with un-trained neural networks: Gradient descent finds the smoothest approximation’, ICML 2020 (International Conference on Machine Learning).
AbstractP. L. Antkowiak, J. Lietard, M. Z. Darestani, M. M. Somoza, W. J. Stark, R. Heckel & R. N. Grass, 'Low cost DNA data storage using photolithographic synthesis and advanced information reconstruction and error correction’, Nature Communications, 2020.
AbstractR. Heckel and P. Hand , 'Deep decoder: Concise image representations from untrained non-convolutional networks’, ICLR 2019 (International Conference on Learning Representations).
AbstractR. Heckel, N. B. Shah, K. Ramchandran, and M. J. Wainwright, 'Active ranking from pairwise comparisons and when parametric assumptions don’t help’, Annals of Statistics, 2019.
AbstractBei Änderungs- oder Aktualisierungswünschen wenden Sie sich bitte an Franz Langer.