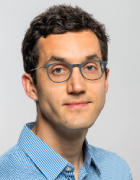
Prof. Dr. Reinhard Heckel
Academic Career and Research Areas
Reinhard Heckel is a Professor for Machine Learning at the Department of Computer Engineering at TUM (Technical University of Munich). From 2017-2019 he was a tenure track assistant professor in the Department of Electrical and Computer Engineering at Rice University. Before that, he was a postdoctoral researcher in the Berkeley Artificial Intelligence Research Lab at the EECS department at the University of California, Berkeley, and worked for a year in the Cognitive Computing and Computational Sciences Department at IBM Research Zurich. He completed his PhD in 2014 at ETH Zurich and was a visiting PhD student at Stanfords University’s Statistics Department. In 2022, he was named as Germany’s “Top 40 unter 40'' by Capital Magazine and was honored by the Werner-von-Siemens Ring foundation for his work.
Reinhard Heckel's research is centered on machine learning and information processing. He focuses on developing algorithms and theoretical foundations for deep learning, particularly in medical imaging application, and on establishing mathematical and empirical underpinnings for machine learning. Additionally, he works on DNA data storage and the utilization of DNA as a digital information technology.
Awards
- Capital 40 under 40 (2022)
- Honor of the Werner-von-Siemens-Ring foundation (2022)
- Featured in the BBC-Future Series ‘The genius behind: This is how to store human knowledge for eternity’ (2015)
- ETH Zurich medal for outstanding Ph.D. thesis (2015)
- IBM first patent application invention achievement award (2015)
Key Publications (all publications)
M. Zalbagi Darestani, Akshay Chaudhari, and R. Heckel, 'Measuring robustness in deep learning based compressive sensing’, ICML 2021 (International Conference on Machine Learning) (long talk).
AbstractR. Heckel and M. Soltanolkotabi, 'Compressive sensing with un-trained neural networks: Gradient descent finds the smoothest approximation’, ICML 2020 (International Conference on Machine Learning).
AbstractP. L. Antkowiak, J. Lietard, M. Z. Darestani, M. M. Somoza, W. J. Stark, R. Heckel & R. N. Grass, 'Low cost DNA data storage using photolithographic synthesis and advanced information reconstruction and error correction’, Nature Communications, 2020.
AbstractR. Heckel and P. Hand , 'Deep decoder: Concise image representations from untrained non-convolutional networks’, ICLR 2019 (International Conference on Learning Representations).
AbstractR. Heckel, N. B. Shah, K. Ramchandran, and M. J. Wainwright, 'Active ranking from pairwise comparisons and when parametric assumptions don’t help’, Annals of Statistics, 2019.
Abstract