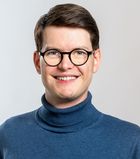
Dr. Vincent Fortuin
Helmholtz AI Young Investigator Group
Efficient Learning and Probabilistic Inference for Science
Professorship of Ethics in Systems Design and Machine Learning
Academic Career and Research Areas
Dr Vincent Fortuin's research focuses on the interface between Bayesian inference and deep learning, with the goal of improving robustness, data-efficiency, and uncertainty estimation in these approaches. While deep learning often leads to impressive performance in many applications, it can be over-confident in its predictions and require large datasets to train. Especially in scientific applications, insights from Bayesian statistics can be used to drastically improve these models. The main research areas include priors and inference in Bayesian deep learning, deep generative modeling, meta-learning, and PAC-Bayesian theory.
Dr Fortuin studied at the University of Hamburg and ETH Zurich, before pursuing his doctoral studies at the Institute of Machine Learning at ETH Zurich, focusing on the choice of priors in Bayesian deep learning. After finishing his PhD, he was a Research Fellow at St John’s College at the University of Cambridge and a postdoctoral researcher in the Machine Learning group. As of March 2023, he is a tenure-track research group leader at Helmholtz AI in Munich.
Awards
- Branco Weiss Fellowship (2023)
- St John’s College Research Fellowship (2022)
- Swiss National Science Foundation Postdoc.Mobility Fellowship (2022)
- Swiss Data Science Center PhD Fellowship (2018)
- ETH Excellence Scholarship (2015)
Key Publications (all publications)
Fortuin V: “Priors in Bayesian Deep Learning: A Review”. International Statistical Review. 2022; 90 (3): 563-591.
AbstractFortuin V, Garriga-Alonso A, Ober SW, Wenzel F, Rätsch G, Turner RE, van der Wilk M, Aitchison L: “Bayesian Neural Network Priors Revisited”. International Conference on Learning Representations. 2022.
AbstractD’Angelo F, Fortuin V: “Repulsive Deep Ensembles are Bayesian”. Advances in Neural Information Processing Systems. 2021.
AbstractFortuin V, Baranchuk D, Rätsch G, Mandt S: “GP-VAE: Deep Probabilistic Time Series Imputation”. International Conference on Artificial Intelligence and Statistics. 2020.
AbstractFortuin V, Hüser M, Locatello F, Strathmann H, Rätsch G: “SOM-VAE: Interpretable Discrete Representation Learning on Time Series”. International Conference on Learning Representations. 2019.
Abstract